The Non-fiction Feature
Also in this Weekly Bulletin:
The Children’s Spot: It’s Probably Penny by Loreen Leedy
The Product Spot: Risk – a strategy board game
The Pithy Take & Who Benefits
Nate Silver, a statistician and the founder of data journalism website FiveThirtyEight, knows that prediction is challenging because it’s where the objective and subjective intersect—this is also why it’s important. As our data-rich world soars past a million terabytes of information, has our capacity to understand, analyze, and make use of the information kept pace? Not really, he concludes, because when humans have “too much information,” we pick out parts we like and discard the rest. The signal is the truth, and the noise is what distracts from the truth.
He examines a wide range of predictive fields (such as economics, weather, earthquakes, gambling, terrorist attacks, the flu) and concludes that the more eagerly we scrutinize our theories and take seriously all the uncertainties hovering around us, the better our predictions will be. I think this book is for people who seek to understand: (1) why uncertainty in predictions is not an enemy to be ignored but to be embraced; (2) why some predictive models fail even though they seem foolproof—wholly objective with ironclad numbers; and (3) how basic predictive equations can be used in everyday life to help make better decisions.
The Outline
Flu
- The epidemiologists interviewed were aware of the limitations of their predictive models. Doctors tend to be cautious; in their field, stupid models kill people.
The Challenges of Prediction
- One of the most useful quantities for predicting disease spread is a variable called the basic reproduction number.
- R0, it measures the number of uninfected people expected to catch a disease from an infected individual. An R0 of 4 means that, without preventative measures like vaccines, someone who gets a disease can be expected to pass it along to four other individuals before recovering or dying.
- Spanish flu: R0 of 3
- Measles: R0 of 15
- (A contemporary note not from the book: COVID’s R0 is roughly 3.5.)
- But, reliable estimates of R0 usually can’t be formulated until well after a disease proliferates. So, epidemiologists must extrapolate from a few early data points.
Weather forecasts
- Although computing power has improved exponentially, progress in the accuracy of weather forecasts has been steady but slow. What makes a good forecast?
- Accuracy: Did the actual weather match the forecast?
- Honesty: However accurate the forecast turned out to be, was it the best one the forecaster was capable of at the time?
- Economic value: Did it help the public make better decisions?
- Calibration: Out of all the times a forecaster said there was a 40% chance of rain, how often did rain actually occur?
- The National Weather Service’s forecasts are very well calibrated.
- The Weather Channel fudges a little: Historically, when they said there is a 20% chance of rain, it only rained about 5% of the time.
- Local networks value presentation over accuracy and honesty.
- This becomes a problem with something like Hurricane Katrina, because many get their weather from local sources.
The Challenges of Prediction
- Chaos theory applies to systems that are: (1) dynamic – the behavior of the system at one point in time influences its behavior in the future; (2) nonlinear – they are generally exponential.
- So, a small change in initial conditions (a butterfly flaps its wings in Brazil) can produce a large and unexpected outcome (a tornado in Texas).
- In forecasting data, even something like a very tiny change in barometric pressure can lead to wildly differing results.
Climate change
- The greenhouse effect—which is the process by which certain atmospheric gases (water vapor, carbon dioxide, and methane) absorb solar energy reflected from the earth’s surface—causes global warming.
- There are three types of skepticism in the climate debate:
- Self-interest. In 2011, the fossil fuel industry spent $300 million on lobbying activities. These companies have a financial incentive to preserve their positions.
- Contrarianism. While some people find it advantageous to align themselves with the crowd, others see themselves as persecuted outsiders.
- Scientific skepticism. These are valid concerns about one aspect of a theory, and to make scientific progress, their points of view must be respected.
The Challenges of Prediction
- There’s little agreement about the accuracy of climate computer models.
- Initial condition uncertainty: Short-term factors compete with the greenhouse signal; the greenhouse effect is a long-term phenomenon, obscured by all types of events on a day-to-day basis.
- Scenario uncertainty: Carbon dioxide circulates quickly in the atmosphere but remains there for a long time; it could take years to reduce the growth rate of carbon dioxide in the atmosphere.
- Structural uncertainty: how well scientists understand the climate system’s dynamics and how to represent them mathematically.
- Uncertainty in climate forecasts compels action, is an essential and nonnegotiable part of a forecast, and has the potential to save property and lives.
Terrorist attacks
- As with Pearl Harbor, 9/11 was supposedly unpredictable.
The Challenges of Prediction
- Humans tend to mistake the unfamiliar for the improbable: The contingency not seriously considered looks strange; what looks strange is thought improbable; what is improbable need not be considered seriously.
- So, perhaps people went through a logical deduction in the 1940s:
- The US is rarely attacked > Hawaii is part of the US > therefore, Hawaii is unlikely to be attacked.
- So, perhaps people went through a logical deduction in the 1940s:
- Unknown unknowns: things people do not know they don’t know. But, there were actually many signals that pointed to 9/11; there was just a failure of imagination:
- There had been a dozen warnings about aircrafts being used as weapons;
- The World Trade Center had been targeted by terrorists before;
- Al Qaeda was exceptionally dangerous and inventive, capable of pulling off large-scale attacks;
- An Islamic fundamentalist named Zacarias Moussaoui had been arrested on August 16, 2001 after he was reported for behaving suspiciously because, even though he was a beginner, he sought training to fly a Boeing 747.
9/11 was not an outlier
- One basic definition of terrorism: the act must (1) be intentional, (2) entail actual or threatened violence, (3) be carried out by non-governmental actors; (4) be aimed at attaining a political, economic, social, or religious goal; and (5) involve some element of intimidation intended to induce fear in an audience beyond the immediate victims.
- This type of terrorism is associated with an increase in attacks against Western countries; from 1979 to 2000 the number of terror attacks against NATO countries rose almost tripled.
- Before 9/11, there were more than 4,000 attempted or successful terror attacks in NATO countries, but more than half the death toll had been caused by just 7 of them.
- The three largest attacks accounted for more than 40% of the fatalities.
- This type of terrorism is associated with an increase in attacks against Western countries; from 1979 to 2000 the number of terror attacks against NATO countries rose almost tripled.
- This type of pattern—a very small number of cases causing a very large proportion of the total impact—is characteristic of a power-law distribution.
- Power laws are important when making predictions about the scale of future risks. They imply that disasters much worse than what society has recently experienced are entirely possible, if infrequent.
- For instance, the terrorism power law predicts that a NATO country experiences a terror attack killing at least 100 people about 6 times from 1979 – 2000 (the actual number is 7).
- It also implies that something on the scale of 9/11, which killed almost 3,000 people, would occur about once every 40 years.
- Power laws are important when making predictions about the scale of future risks. They imply that disasters much worse than what society has recently experienced are entirely possible, if infrequent.
TV Politics
Hedgehogs and Foxes
- The more interviews an expert does with the press, the worse the overall predictions.
- There are hedgehogs and foxes (from Leo Tolstoy’s The Hedgehog and the Fox). Foxes know many little things, and hedgehogs know one big thing.
- Hedgehogs are type A personalities who believe in Big Ideas that govern the world as though they were physical laws. (Like Karl Marx and class struggle.)
- Foxes believe in lots of little ideas and take a lot of approaches toward a problem. They’re more tolerant of uncertainty, complexity, and dissent.
- Foxes are better at forecasting. For instance, they had more closely predicted the Soviet Union’s fate. Rather than seeing the USSR ideologically (evil, etc.) they saw it as an increasingly dysfunctional nation.
The Challenges of Prediction
- Hedgehogs make better TV guests. And, the more facts hedgehogs have, the more opportunities they have to manipulate them to confirm their biases.
- Foxes have trouble fitting into type A cultures like TV, business, and politics. They believe that many problems are hard to forecast, but this may be mistaken as a lack of self-confidence.
- Important political news proceeds at an irregular pace. But news coverage is produced every day. Often, its presentation obscures its insignificance, which heightens the noise.
Economists
Economic forecasts generally
- Economic forecasts rarely anticipate economic turning points more than a few months in advance, and can fail to predict recessions even when they’re already happening.
- In 2007, economists in the Survey of Professional Forecasters thought a recession was relatively unlikely to happen.
- Most of their predictions do not come to pass.
- Studies from another panel, the Blue Chip Economic Survey, have more often come up with accurate findings.
- The former is conducted anonymously, but the latter is not.
- This is the “rational basis” phenomenon.
- The less reputation an economist has, the less there is to lose by taking a big risk, even if the forecast seems shady.
- If an economist is already established, there’s more reluctance to step too far out even if the data demands it.
- These both probably worsen forecasts on balance.
The Challenges of Prediction
- Economic forecasters face three fundamental challenges:
- It’s very hard to determine cause and effect from economic statistics alone.
- The economy’s always changing, so explanations of economic behavior in one business cycle may not apply to future ones.
- As bad as their forecasts are, the data the economists have to work with isn’t very good either.
The 2008 Recession
- The housing bubble and stock market crash were catastrophic failures of prediction, where key players ignored hard-to-measure risks despite the threats they posed.
Ratings Agencies
- The ratings agencies (companies that assign credit ratings on debt) gave their highest rating, AAA, to thousands of mortgage-backed securities (which let investors bet on the likelihood of someone defaulting on their home).
- For instance, rating agency Standard & Poor rated collateralized debt obligation, an extremely complex security, at AAA, with only a 0.12% probability of default. Ultimately, 28% defaulted.
- The ratings agencies blamed the housing bubble and said “nobody saw it coming.”
Housing Bubble
- Many saw the housing bubble coming and said so well in advance.
- The 2000s had record-low rates of savings, but mortgages were extremely easy to obtain—thus, prices became untethered from supply and demand.
- Home prices decreased in 17/20 of the largest markets, and the number of housing permits declined (a leading indicator of housing demand).
The Ratings Agencies and the Housing Bubble
- Imagine a stranger wants to sell a woman a car and a friend vouches for the stranger, so it’s more likely that she’ll buy. The ratings agencies would have been that friend, vouching for mortgage-backed securities using AAA ratings.
- The ratings agencies should have been the first to detect problems. They had the best information on whether people made timely mortgage payments.
- Instead, ratings agencies took advantage of their status to build up exceptional profits—the more collateralized debt obligations, the more profit.
The Challenges of Prediction
- There were four major failures of predictions, caused in large part because each failure was “out of sample.”
- For example, say a person’s a good driver and has driven 20,000 trips. One night he gets wasted and thinks, well, all those trips were fine, so this one will be fine.
- But, his sample size for drunk driving isn’t 20,000, it’s zero, so he can’t use past experience to forecast risk. His prediction is out of sample.
- For example, say a person’s a good driver and has driven 20,000 trips. One night he gets wasted and thinks, well, all those trips were fine, so this one will be fine.
- First, homeowners and investors thought that rising prices implied that home values would continue to rise, even though historical data implies this usually leads to decline.
- Out of sample: There had never been such widespread increase in US housing prices like the one before the collapse.
- Second, the ratings agencies failed spectacularly, as their forecasting models were full of faulty assumptions and false confidence about the risk of a housing collapse.
- Out of sample: The ratings agencies hadn’t rated such novel and complex securities before.
- Third, there was widespread failure to anticipate how a housing crisis could trigger a global financial crisis.
- Out of sample: The financial system had never made so many side bets on housing before.
- Fourth, policymakers and economists failed to predict the scope of the economic problems, thinking the economy could rebound.
- Out of sample: Prior recessions hadn’t been associated with financial crises.
- Forecasters often resist considering out-of-sample problems because it forces them to acknowledge that they know less about the world than they thought.
Thomas Bayes
- The author references and utilizes Bayesian reasoning multiple times.
- Bayes was an English minister born in the early 1700s and created a thought process to govern decision-making by regarding rationality as a probabilistic matter.
- It’s just an algebraic expression with three known variables and one unknown one.
- Bayes’s theorem deals with conditional probabilities. That is, it tells us the probability that a theory is true if some event has happened.
- For example, say a woman returns home from a trip and she finds a strange pair of underwear on her partner’s dresser. What’s the probability that the partner is cheating?
- The condition: she found the underwear.
- The hypothesis: the probability that she’s being cheated on.
- First, she has to estimate the probability of the underwear appearing as a condition of the hypothesis being true. Let’s say she puts the probability of the underwear appearing, conditional on cheating, at 50%.
- Second, she needs to estimate the probability of the underwear appearing conditional on the hypothesis being false. What if they were a gift? She puts this probability at 5%.
- She also needs a prior probability: What’s the probability she would have assigned to the partner cheating before finding the underwear? Studies have found that about 4% of married partners cheat, so assume that’s the prior.
- When inserted into the equation, the probability is fairly low: 29%.
- But, isn’t the underwear pretty incriminating? The low percentage stems from the low prior probability (4%) assigned to the partner cheating.
- When people’s priors are strong, they’re surprisingly resilient in the face of new evidence.
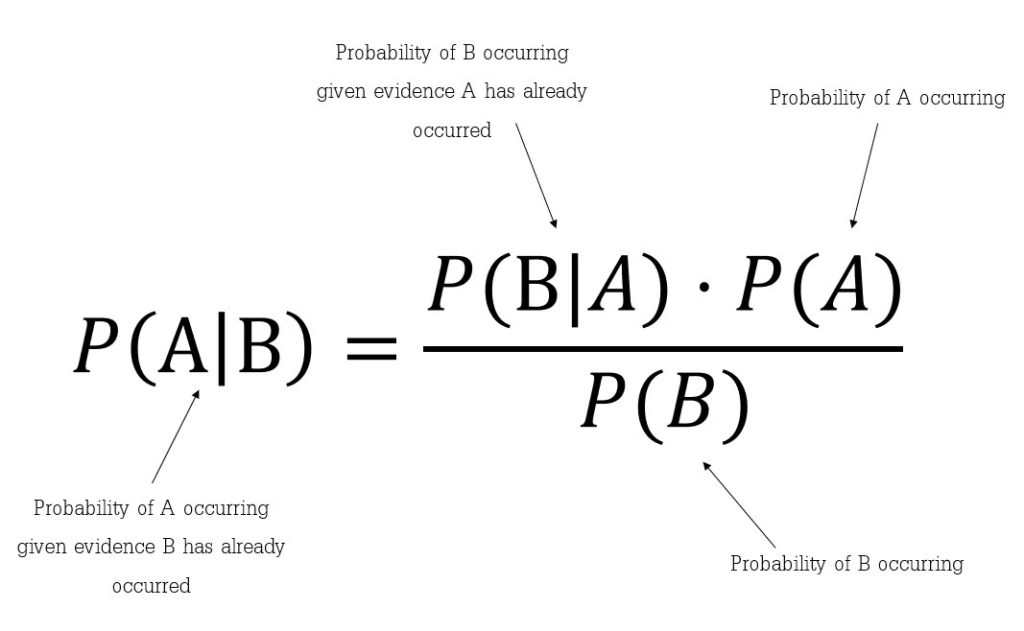
- The Bayesian approach is especially helpful for decision-making under high uncertainty. It encourages people to hold a large number of hypotheses at once, to think about them probabilistically, and to update them frequently with new information.
And More, Including:
- Statistics, Moneyball, and how the information revolution transformed baseball using the sport’s unique combination of technology, competition, and rich data
- How earthquakes cannot be predicted but can be forecast, and what we know about how earthquakes behave
- How gamblers and poker players think: finding patterns is easy in any data-rich environment, but determining whether the patterns represent noise or signal is hard
- Why a bug in IBM’s DeepBlue rattled chessmaster Garry Kasparov
- Why people can’t fully prevent the herd behavior that causes financial bubbles and why it’s nearly impossible to “beat the market”
The Signal and the Noise: Why So Many Predictions Fail – But Some Don’t
Author: Nate Silver
Publisher: Penguin Books
Pages: 576 | 2015
Purchase
[If you purchase anything from Bookshop via this link, I get a small percentage at no cost to you.]
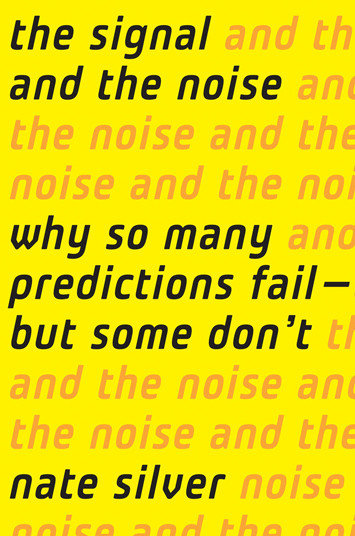